How to get to market with Machine Learning
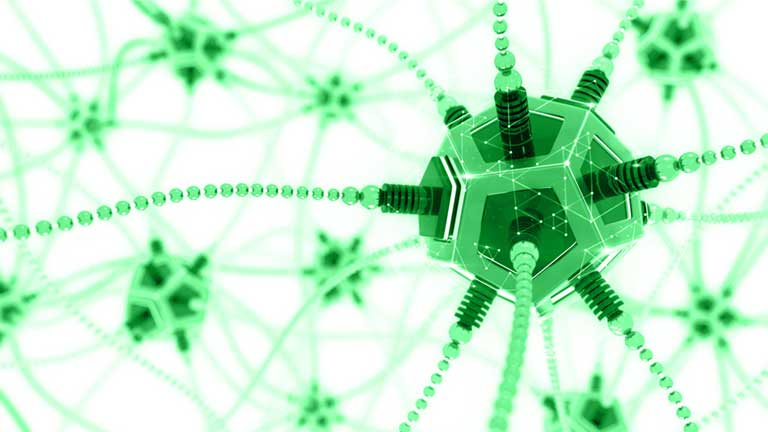
Some may think of Machine Learning as the supporting act for AI, but in the industrial sector it is the main attraction.
Artificial Intelligence (AI) will become so commonplace it will be taken for granted. We can say this with some confidence, because so many semiconductor manufacturers already have embedded processors that are designed for AI. These devices will become as ubiquitous as the current technology workhorse: the microcontroller. In fact, they are the natural evolutionary step for the MCU.
This poses the very relevant question of how to get started with AI. But this ignores the answer to a more relevant question, which should be “what kind of AI is right for you”. Yes, AI will be everywhere, but it isn’t a panacea to design. Engineers will still need to choose the best solution for their application.
By understanding the use-case, the possible solutions often suggest themselves. That was true before AI became so accessible and it remains true now. In that respect, AI is like any other component.
What has changed, however, is what a customer looks like. AI emerged in the software domain, so it follows that the real experts – those with 10 years or more of experience – are software companies. Getting that software to market, particularly in verticals like industrial, medical, and aerospace, takes the right hardware.
Whilst Machine Learning (ML) is being used in multiple use-cases, including preventive maintenance. it is vision that is attracting the greatest interest. This could include an application for Smart Cities, where cameras are being used to detect activities, such as using a mobile phone while driving.
Choosing the right hardware for industrial vision with ML
When developing a machine vision system, selecting the right image sensor could be one of the most important design decisions. This is even more relevant when ML is going to be used to analyze the image data.
If you want to use machine vision with ML to check something fast-moving, like a bottle on a production line, you need to use a camera module with a global shutter. A rolling shutter would not work in this application, because there is a chance that the details will be missed.
Similarly, the image sensor needs to be appropriate for the operating environment. In some applications it will be necessary to select an image sensor that also has the right low-light performance. These considerations exist when using machine vision, but they can become more relevant when generating data that will be interpreted using ML.
Because the data is being analyzed by an algorithm and not a person, this presents the opportunity to introduce different sensing modalities in machine vision systems. This may include modals other than image sensors, such as time of flight, radar and lidar. With this comes the need for sensor fusion.
Sensor fusion is the process of taking data from multiple sources and combining it into a single set that can be fed into a neural network. It is a crucial stage, because the way each sensor’s data is weighted will influence its relevance on the final data set.
Training and transfer learning for ML in industry
Whilst creating a demonstrator to prove the concept of machine learning is not as difficult as it used to be, but taking the concept through to production can be a challenge.
Standard data sets can be used for demonstration purposes, but often those standard data sets will not be suitable for implementation. Customers may need to buy data from companies experienced in creating data, or they need to create it themselves.
This is where techniques such as transfer learning can be useful, which takes data created for one use-case and ports it across to a new but similar use-case. But this is only one solution. Another may be to create synthetic data virtually, using 3D modelling. This allows many images to be created quickly, which can be used to train a machine learning algorithm without the effort or expense of physically creating examples.
Edge versus the Cloud
This is a question that comes more often now that AI is moving to the network’s edge, but the two will continue to coexist, because each can offer benefits the other cannot.
For example, if security or latency are a particular need in the use-case, then edge processing may be appropriate, but if the application demands an algorithm that is capable of continuous improvement, then processing in the cloud could be a better solution.
It can make sense to use both edge and cloud. If you have many products in different locations, such as a smart thermostat, then processing at the edge can be used. But sharing the data through the cloud could lead to improvements in the algorithm, which could be rolled out across the entire network of thermostats.”
Conclusion
The use of machine learning in in the industrial sector is increasing, thanks in part to increased access to high-performance processors equipped with powerful AI acceleration technology. But the software remains a key part of the equation, both in training and deployment. Modelling techniques and transfer learning can help, but so too can the resources and software tools available from processor manufacturers. Hardware and software must go hand-in-hand to develop a successful use case with machine learning.
Avnet and its partners are well positioned to help you understand how to get to market using machine learning. If you would like to find out more, you can connect directly with our AI/ML experts here.



